In the wake of the Biden administration’s“Executive Order on the Safe, Secure, and Trustworthy Development and Use of Artificial Intelligence,” industry and academic leaders in machine learning and artificial intelligence have been busy digesting and interpreting its implications for tech at large. But the discourse over “responsible AI” is nothing new to Columbia Business School's Digital Future Initiative (DFI).
Earlier this fall, DFI conducted a workshop that brought together some of the leading experts on operationalizing responsible AI. Hosted by Omar Besbes, the Vikram S. Pandit Professor of Business, and organized by Assistant Professor Hongseok Namkoong, the event included thought leaders from Harvard, MIT, LinkedIn, and Meta, among others.
Namkoong is an interdisciplinary scholar working at the interface of AI and operations management. CBS spoke with Namkoong to discuss his takeaways from the workshop and what responsible AI means — both today and for the foreseeable future.
CBS: How did you become interested in responsible AI?
Hongseok Namkoong: My particular field is sort of a nascent topic — a research area, by and large. Its connection to topics like responsible AI is this: If you try to apply AI models in a high-stakes domain, like a large business, you soon realize that you can’t always trust the output of an AI – that it can silently fail in unexpected ways. It doesn’t fully grasp the extent of what it doesn’t know.
All of this goes under slightly different names, like robustness, fairness, causality, and so on. And all these different rules that we encode in AI are learned from its training data. You could think of data as a byproduct of the socioeconomic system that we’re operating under.
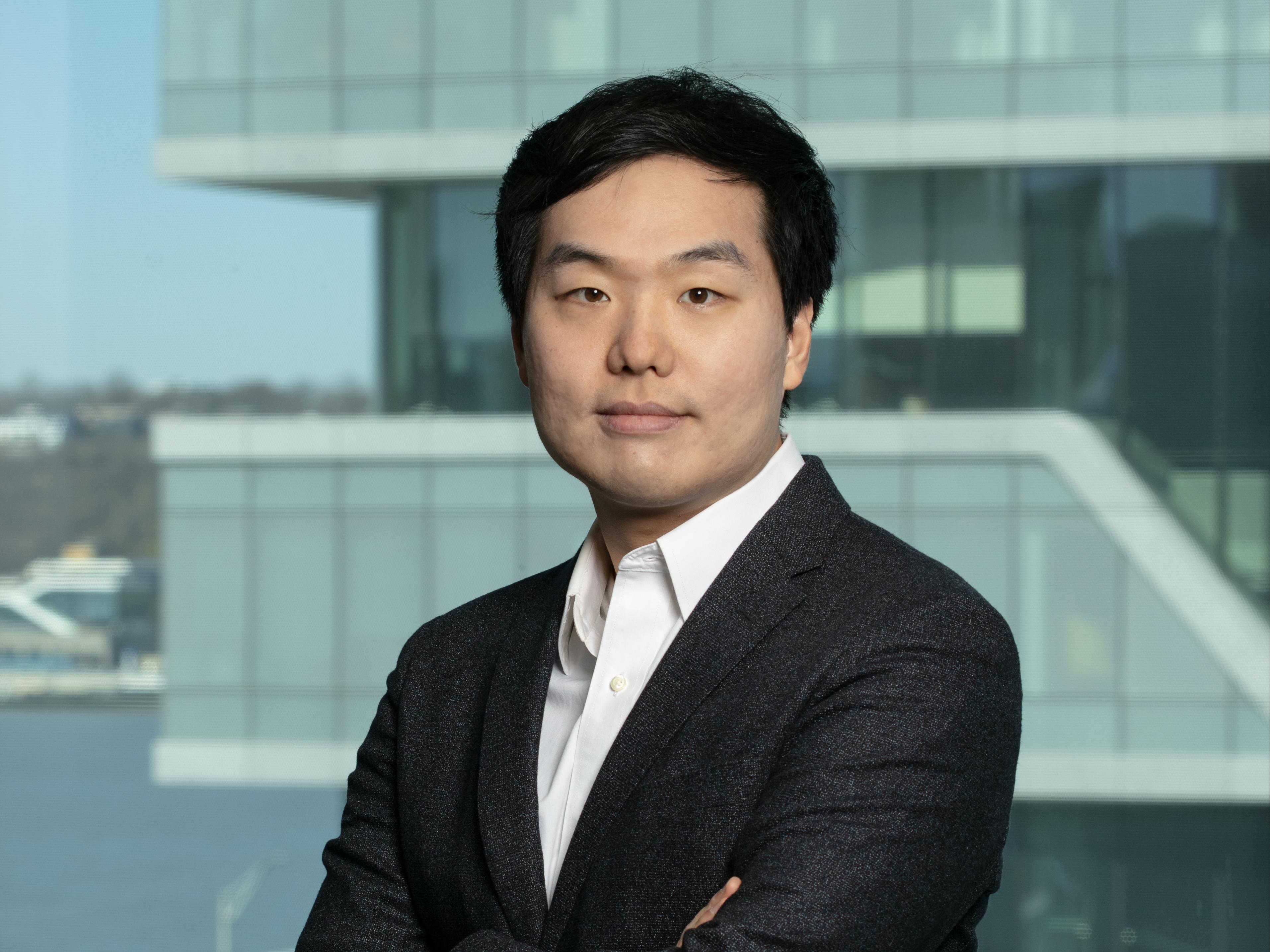
CBS: That raises the question of the purity of the data. Is there bias inherent in the data that the AI model itself is trained on? Are there privacy concerns — even ethical concerns — in terms of how the data set has been collected or scraped without someone’s consent?
Namkoong: Like any topic, technology does not live in a vacuum, and it's contingent upon the social context in which it was developed and used. So, in some sense, these data sets all embody the capital interests and the social relations of the world that we live in. The same applies to all the models that we end up building off of this infrastructure. A lot of researchers have started adopting this concept of “infrastructure” because they're very much like roads and sewers in the sense that once they're established, it's really hard to change. So any models trained off of data are going to essentially reproduce and replicate the power structures that we see in society.
In practice, every data set is biased. We live in a society full of structural racism. Any model we develop in that context will have these given limitations. To me, then, the problem before us is that we need to develop a language to capture that — either in a legal sense, a regulatory sense, a socially conscious sense, or a corporate sense. How are we going to articulate these biases and mitigate them? Some of it is quite straightforward — and some of it really isn't.
CBS: What is CBS doing to advance the development of responsible AI?
Namkoong: CBS is pushing the envelope in providing a community in which we can have a grounded discussion on what it means to implement responsibility practices. What are the best practices, and what are the key challenges we're facing? All of these efforts don't happen in a vacuum, right? There are all kinds of vested capital interests that are interfering or facilitating these endeavors. There's different types of regulatory pressures and compliance topics that corporate firms need to think about. With the AI workshop, I wanted to bring together folks who are on the ground trying to do things within their organizational context, under resource constraints. You could say that this is part of my identity as an interdisciplinary AI researcher: Resource constraints are something we too often ignore in AI academia, although a central focus of operations management.
CBS: Ideally, we’d have responsible AI by the end of the week. But realistically, how quickly can that happen? Does the pace of integrating it depend on how a company is using AI and how quickly it can scale? It seems like that would differ across industries, from organization to organization.
Namkoong: Exactly. It's also dependent on the extent to which interests are aligned. So a company like LinkedIn cares deeply about this, even at the C level, because it's a professional network. Incentives are extremely aligned in terms of LinkedIn's ability to handle AI with care and responsibility, and the trustworthiness of the platform is integral to its business model. But for other platforms, you can imagine the extent to which responsible AI is a central topic can vary substantially.
CBS: Do you think even the definition of responsible AI will have to be continuously revisited because of the nature of this technology and how rapidly it’s evolving?
Namkoong: For sure, I don't think there is a definition that people agree on. I don't think there is a definition that I even agree on in a consistent way. I mostly think of it as caring about optimal decisions. We have the current status quo. We need to be able to take gradient steps towards a better equilibrium. So, in that sense, what are the more responsible practices we can institute and operationalize, and what are the different parties that we can move to get buy-in? That’s often how I think about these things.
CBS: Judging by your workshop’s panel, one of the first steps for any organization would be to figure out how to actually audit its operational use of AI, to see how it compares to industry standards and benchmarks.
Namkoong: Right. And that audit comes in multiple layers. One is compliance. What is the current legislation saying about the bare minimum that a company should be doing? For example, this is fairly well defined in banking. The commercial banks for decades have been subject to these laws that say that whenever you deny an applicant a loan, you're responsible for giving them some information on why they were rejected. And your practices really cannot be discriminatory. So then a lot of these AI lending startups, or even the largest commercial banks like Chase, have these AI teams in charge of compliance of their AI models. Relatively speaking, the legal landscape is fairly well known and established in operationalizing.
CBS: What would you want people to know about machine learning and AI that has gone largely ignored in all the discourse?
Namkoong: My sense is that the set of people who are actually making progress in AI is a little bit separate from the people who are engaging in these “doomer debates.” I have never seen a productive debate on that topic where anyone has walked away with a deeper understanding of the space than they had before. That's why I intentionally chose to focus on operationalizing best practices in this workshop. I feel like that's something that's really difficult to do, particularly given a high-interest-rate environment with resource constraints.
How do I convince my boss's boss to assign more resources so we can develop better best practices that allow us to operate in a more responsible way? That's not an easy thing to do, and it comes with a whole lot of different nuances. That's something that our MBA population would be excellent at doing.